Biomarker discovery 2.0: Using new technologies to access novel insights into human health and disease
Due to the size and dimensionality of the datasets required, data science is an essential component of modern biomarker discovery and development. It's no longer feasible to mine and process these data sets manually, which demands collaboration between R&D specialists, bioinformaticians, and data scientists.
However, this also presents new opportunities. New technologies like next-generation sequencing (NGS) and methods such as machine learning can allow us to discover more complex and fine-grained patterns in the data than was previously possible.
The impact of NGS & multi-omics technologies on biomarker discovery
Advances in NGS and multi-omics technologies have fundamentally transformed the landscape of biomarker discovery, revolutionizing the way researchers investigate and understand complex biological systems. The scope and resolution of data generation technologies has long been - and will continue to be - a limiting factor for biomarker discovery. Methods like ELISA and Western blotting were low throughput, restricting comprehensive analysis. Moreover, these techniques often targeted specific biomolecules, hindering discovery of novel markers and hampering integration across molecular layers. Long turnaround times, challenges in data integration, and limited sensitivity further impeded progress.
The advent of NGS has revolutionized biomarker discovery, overcoming these constraints and enabling more comprehensive and accurate analyses. Researchers have gained unprecedented access to high-throughput sequencing, enabling the rapid and cost-effective analysis of entire genomes, transcriptomes, proteomes, metabolomes and epigenomes. Moreover, the integration of multi-omics approaches has provided researchers with a more comprehensive view of biological systems.
The power of multi-omics analysis lies in the multi-layered nature of the datasets. By analyzing multiple molecular layers simultaneously, researchers can elucidate complex interactions and identify biomarkers that may not be apparent from individual datasets alone. This integrative approach enables researchers to capture more of the complexity of biological processes, leading to the discovery of novel biomarkers with improved specificity and sensitivity.
Furthermore, NGS and multi-omics technologies have catalyzed the shift towards personalized medicine by facilitating the identification of biomarkers associated with individual variability in drug susceptibility, disease progression, and prognosis. By analyzing the molecular profiles of individual patients, researchers can tailor therapeutic interventions to target specific molecular pathways or genes, ultimately improving patient outcomes. This systems-level understanding of disease biology has the potential to transform the development of precision therapies, leading to more effective treatments for a wide range of diseases, such as cancer and neurodegenerative conditions.
A key barrier to leveraging the potential of multi-omics analysis to drive better, faster biomarker discovery has been a lack of tools that allow biomedical domain experts to deal with these massive and complex data resources. It is not feasible for all biomedical scientists to also be experts in data science - and thereby tools that allow life scientists to easily explore and analyze their multi-omics data through intuitive, user-friendly interfaces are essential. Only then can R&D teams easily extract actionable information to form data-driven, testable hypotheses that drive forward biomarker discovery.
Our answer to this challenge is Bio|Mx: a pioneering multi-omics integration solution that combines public and proprietary omics datasets to provide you with comprehensive overviews of biological systems in a disease context.
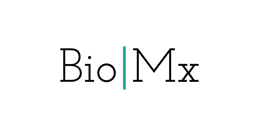
Find out more about Bio|Mx here.
The impact of AI and ML on biomarker discovery and development
Due to the large amounts of complex biomedical data now routinely generated in the field of biomarker development, artificial intelligence (AI) and machine learning (ML) techniques have become essential for data analysis. These technologies provide a holistic approach to data integration and analysis, accommodating diverse sources like genomics, transcriptomics, proteomics, metabolomics, and clinical data.
The power of AI/ML algorithms lies in their capacity to process large multi-omics datasets with unmatched efficiency. They excel at identifying complex patterns, correlations, and associations across different molecular layers that traditional analytical methods might miss. By combining molecular profiles with clinical data, AI/ML models can reveal potential biomarkers that are important in understanding disease onset, susceptibility, progression, and treatment response. This sets the stage for improved risk scoring and more personalized medicine.
Furthermore, AI/ML techniques can aid healthcare professionals in making clinical decisions. These systems generate personalized treatment recommendations by integrating patient-specific information, such as genetic variants, biomarker levels, and clinical history. By employing AI/ML-driven risk scoring models, clinicians could also stratify patients based on their disease risk and tailor interventions. This results in optimized patient outcomes and resource allocation.
What are the benefits & pitfalls of using machine learning for biomarker discovery?
ML-based biomarker discovery can also present several challenges and pitfalls. One notable concern is overfitting: capturing noise or spurious correlations in the data, leading to poor generalization performance on unseen datasets. Addressing overfitting requires strong testing methods and thoughtful choice of model settings to guarantee reliability and repeatability of biomarker results - in other words, it’s important to have experts who understand both data science and the biological context on your team.
Moreover, ML algorithms may encounter issues with dataset bias or imbalances, particularly when working with heterogeneous or underrepresented populations, which could lead to biased biomarker predictions or limited generalizability across diverse patient cohorts. As we’ve discussed in previous blogs, for this reason it is essential to choose datasets that match the biological questions you have, and to meticulously curate and annotate those datasets. AI can be used to assist this process, but human curation remains essential to achieving high-quality datasets - and thereby high-quality ML-empowered results.
Another challenge is the interpretability of ML models. Many models are built as “black boxes”: so although they may provide interesting predictions based on biomedical data, they fail to provide insight into how and why the algorithm made that prediction. When used in biomarker discovery R&D, this makes it difficult to understand the underlying rationale behind biomarker predictions. Interpretable ML methods and model-agnostic techniques can help mitigate this issue by providing insights into feature importance or decision processes, enhancing the trustworthiness and usability of biomarker discovery results.
Do you want to build a “white box”, explainable model to support you in biomarker discovery?
BioLizard has proven expertise in leveraging explainable AI, so that your domain experts can challenge and understand the results, and feed the knowledge gained back into your scientific trajectory.
Conclusion
Leveraging new tools and technologies such as ML can enhance the biomarker discovery and development process, facilitating better decision-making and enabling researchers to identify promising candidates more effectively. However, the use of new tech must remain biology-centric: starting from your biomedical questions and your data, and relating every AI decision to those. At BioLizard, we aim to build the connections between your data, AI, and your unique research goals - customizing our approaches to match your needs to provide you with biology-centric, curated insights that drive your R&D forward.
Ready to experience the BioLizard difference?
Reach out to us now to start discussing how we can support you.