Statistical challenges faced when analysing single cell sequencing data
Throughout recent years, single-cell sequencing has evolved into a multi-purpose toolkit that tackles various biological questions. However, it’s a toolkit that generates data. A lot of data. More data means more information to process: causing unique challenges during the analysis.
Different omics approaches come with their own unique analytical challenges. That being said, during previous single-cell projects with clients, we’ve noticed some recurring challenges. To help you during your single-cell projects, we’ll happily share them with you.
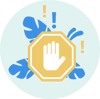
Risks when conducting single-cell research
Single-cell sequencing allows you to dive deeper into the biology of cells and research them on an individual level. This generates more data to process. To put things in perspective: bulk sequencing technology captures the average of a mixture of cells. Single-cell sequencing, on the other hand, can capture the transcriptome of up to 20 000 single cells. This implies a 20 000 fold increase in information to process, and this only considering transcriptomic data!
This increase in data creates some challenges, like:

Computational challenges
Do you have the processing power? Or the storage? Depending on the size of the dataset, you’ll need 32 or up to 64 GB RAM and enough storage (sometimes up to 1TB). On top of that, it could be smart to have multiple CPUs to speed things up. We doubt the average office laptop could handle these requirements.

Challenges with analytics
One thing is that it gets harder to pinpoint what’s important and what’s not when you have more information. Another thing is the risk of using the wrong statistical assumptions, which form false conclusions. As opposed to bulk sequencing methods, workflows for single-cell analysis are complex. It requires a certain set of statistical skills to identify the right approach.

Challenges with experimental design
The experimental and computational departments often function as two separate entities within an organization. Both parties should interact and collaborate to obtain most of your single-cell sequencing data: starting from the experimental design! Regardless of the biological question that you might want to ask, computational and wet-lab professionals are there to help you build an approach to avoid lacking statistical power in the analysis.

Challenges with interpretation
A large amount of data also makes interpretation difficult. Once data is processed, cleaned, filtered, and suitable analytical algorithms have been applied, how can someone correctly interpret this information? The (bio)-informatician – while capable in coding and analytics – often lacks the deep knowledge into the biological matters provided by researchers.
A good starting point to deal with most of these problems comes from thinking about the correct statistical setup before starting. Every research project is different and needs a unique approach.
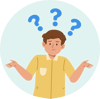
Uncertainty
Single-cell sequencing methods can generate information from hundreds to even thousands of cells. A problem that comes up within single-cell sequencing is the uncertainty regarding observations.
With single-cell sequencing, the amount of input material per cell is low. Due to technical limitations, researchers are faced with measurement uncertainty and missing data. It is important to detect and quantify uncertainty and prevent it from growing in downstream statistical analyses. We hate to break it to you, but there is no “one” methodology or approach that suits all scenarios. Each experiment has its own unique properties, which needs a unique approach.
Another example when dealing with uncertainty in processing data from single-cell experiments resides in the data analysis itself. For example, in single-cell RNA sequencing, one of the usual steps is to perform unsupervised clustering. This method enables the identification of the cell type of each cell in the dataset. Assigning a type to a group of cells is an ad-hoc decision you need to make. It introduces uncertainty that can again grow and affect the downstream analyses.
How to deal with uncertainty?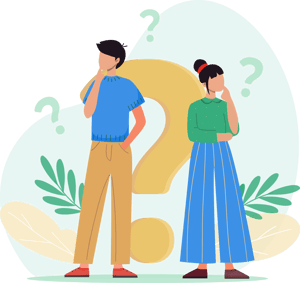
The question is: can you account for the uncertainty? The answer is yes and no. In statistics, it is crucial to be aware of these scenarios and at least try to detect, quantify and correct (if possible) uncertainty.
Luckily, there are methods designed to deal with most uncertainty-related challenges in single-cell experiments.
We’ll happily share them with you:
- Data integration across different samples or experiments (e.g. pseudo-bulk analyses).
- Validating results using publicly available datasets.
- Incorporating expert knowledge. Often researchers or biologists already have a decent understanding of what is expected. Using this knowledge to make the right assumptions or validate approaches is key.
- Applying normalization and/or batch correction to your data to remove unwanted sources of variation.
- Taking your time to think about the statistical design, make power calculations, and determine minimal criteria beforehand (for example, number of cells, purification %, …).
- Exploring your data using basic statistical and visualization techniques to find technical sources of variation.
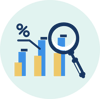
Understanding your results
Processing the data is one thing, but when you analyse hundreds of thousands of cells: how do you keep an overview? Visualization tools are essential for single-cell projects. They provide understandable ways to visualize and analyze single-cell datasets for the users without any computational knowledge.
Creating a clear overview of results isn’t only great for researchers. It also is a major bonus when you need to present results to management or other parties who might lack time and/or more profound scientific knowledge. At BioLizard, we use an open-source visualization tool for single-cell RNA sequencing datasets: cellxgene.
What’s the take-away here?
We have touched upon some of the challenges we face when working with single-cell sequencing data. These challenges relate to data processing and analysis, dealing with uncertainty, and visualising results. In short, the answer to them lies within adequate and tailored data analysis, this way you’ll get the most out of your single-cell dataset.
Keep in mind that there are more challenges to look out for. We didn’t go through them since they are technology-specific.
It’s not a surprise that single-cell sequencing methods generate more complex and richer datasets as compared to bulk sequencing. This higher complexity means more room for misinterpretations and deriving wrong conclusions.
Looking for an experienced partner in single-cell sequencing projects?