Multi-omics analysis: Why and when to go beyond single omics?
From basic research, to drug and target discovery, to clinical phase studies, the life sciences can benefit greatly from the use of multi-omics analysis - when it's used strategically.
Multi-omics analysis can help you build a more complete biological understanding
While single omics studies are powerful to be sure, by definition they miss patterns and interactions that are truly multi-modal, spanning across the DNA, epigenetic, RNA, and protein levels. Adding in additional layers of omics data allows us to untangle these interactions and relationships, resulting in both scientific and financial advantages.
In other words, multi-omics analysis can provide a unified snapshot of the biological processes within a cell or tissue in a manner beyond what any single omics dataset can capture. This clearer, more complete overview of biological processes that multi-omics analysis provides can help identify important players and interactions that may be missed with single-omics analyses, as exemplified by the fact that multi-omics analysis can predict outcomes, such as disease progression, better than single omics analysis. This then translates into an economic advantage: by using multi-omics analysis, you reach a high resolution in one shot. Depending on your scientific question, using multi-omics analysis can get you to the same scientific insights quicker, translating into less time and money spent repeating experiments over the course of the project. On the other hand, you should also be aware that multi-omics projects require skilled expertise, and still will need some time and financial input, to get the most out of analyses.
So, let’s dive into some examples of how using multi-omics analysis can bring your life science research to a new level of clarity, and allow you to access novel biological insights. We’ll start with a case study in which each single-omics dataset provided a biological story that became clearer and more complete upon integration…
Case study 1: Multi-omics analysis provides improved biological understanding of chronic disease onset and progression
Aim: Identify the molecular origin of a chronic degenerative disease, identify biomarkers that can detect early onset, and pinpoint potential drug targets.
Current situation:
- The client’s research focuses on a chronic degenerative disease. While treatments can ease symptoms and slow progression of the disease, the condition cannot be cured.
- Tissue damage can occur well before clinical symptoms appear, providing a time window to early detect disease onset before considerable injury is provoked.
- The client has a large collection of single-omics datasets, including whole genome sequencing, transcriptomics, and proteomics data, derived from multiple different clinical trials investigating patients at different stages of disease progression.
- The molecular mechanism responsible for the onset of the disease is still not clear, and thereby the client would like to integrate these historical datasets to see if a clearer biological picture can be achieved.
BioLizard strategy & process:
- BioLizard performed “longitudinal” integration per data type (e.g. protein or mRNA) with studies as batches, and taking into account the tissue from which the data originated, as well as “vertical” integration, where multi-omics read-outs from the same tissues were merged together allowing for multi-modal analysis.
- Following quality control, longitudinal integration highlighted which samples across studies were outliers that could be removed to reduce technical noise.
- Data were analyzed in order to clarify early markers of disease prior to appearance of clinical symptoms, markers associated with disease progression or worse outcomes, and markers associated with beneficial effect of existing therapeutics.
Results:
- Results were highly consistent across clinical trials and studies in animal models, and dysregulation of known disease-associated pathways was present as expected.
- Multi-omics integration provided higher resolution for identification of molecular changes in relevant tissues during disease onset, uncovering potential biomarkers for disease onset and progression.
- Multi-modal integration revealed key factors in the immunological response associated with disease progression, which were not apparent in single-omics datasets - including cell types and metabolic changes involved in driving disease progression.
In summary:
- A more complete biological picture: Integration of multi-omics data derived from multiple in-house datasets provided new, more detailed insights into features driving disease onset and progression, and identified novel biomarkers.
- Actionable insights: Client received a clear relational overview of different factors underlying disease onset and progression, a detailed report clearly explaining data processing and analysis strategies, and actionable insights to further investigate in follow-up studies.
If you don’t have a bank of in-house data like this client did, never fear! Use of public datasets is a great way to further improve the efficiency of multi-omics analyses. Another client case of ours presents a good example of how the use of public data can benefit target and biomarker discovery…

Case study 2: Integrating public multi-omics datasets to elucidate mechanism of action
Aim: Leverage publicly available datasets in order to deconvolute the mechanism of action underlying disease progression.
Current situation:
- The client would like to perform a proof of concept (PoC) study to assess the potential for using multi-omics data to identify drug target candidates and biomarkers, in a specific disease area of interest.
- A list of public datasets was delivered by the client for this purpose.
- The disease of interest has different sub-classes, complicating data integration and analysis.
BioLizard strategy & process:
BioLizard (re)analyzed and integrated 25 publicly available multi-omics datasets, including transcriptomics, proteomics and epigenomics datasets derived from a range of different tissues. The workflow included:
- Performing preprocessing & quality control for each individual public data set,
- Upon positive evaluation, performing a second step of tissue-wise dataset integration within each -omics where possible,
- Performing dimensionality reduction, differential gene analysis, and gene enrichment analyses in selected datasets that passed quality control, and
- Integrating data encompassing different omics, to provide a more comprehensive biological picture of the disease and its progression.
Results:
- Some datasets failed quality control checks and were eliminated prior to integration.
- Integration of RNA-seq and microarray datasets respectively provided potential insights into the role of genes associated with the disease of interest and with its progression, and combining multiple public omics datasets increased resolution power.
- This data integration demonstrated that integrating high quality, compatible datasets provides added value that is more than the simple sum of the insights possible to obtain from single studies alone.
- BioLizard delivered processed datasets, in-depth reporting with thorough explanation of all methods utilized, publication-ready graphics visualizing the biological insights achieved, and actionable insights that support further integration and in-depth analysis of additional high quality datasets.
In summary:
- Use of public data: using publicly available datasets permitted rapid assessment for the relevance of tissue-specific multi-omics datasets in this use case
- Quality control and biological knowledge are essential: biologically relevant insights were achieved by careful vetting of datasets for inclusion using a combination of bioinformatics skills and biological understanding, which will ultimately support data-driven drug development and biomarker identification
- Actionable insights: the PoC study demonstrated the potential for high-quality multi-omics data to identify target genes and biomarkers for the disease of interest. In the future, additional high-quality datasets can be integrated and leveraged to identify (additional) drug target candidates and biomarkers.
This case also highlights how having an expert partner can provide an advantage for the complex steps of data quality control and integration. In the case of multi-omics analyses, data integration in particular presents some significant bioinformatic challenges. We’ll get into what data integration in the context of multi-omics studies really means, as well as some best practices for multi-omics analysis, in the next blog in this series.
Opportunities & challenges with multi-omics analysis
Although you might expect a one-to-one correlation between gene expression & protein abundance, it’s not always the case. Even if levels of a given mRNA transcript increase, protein content might not increase accordingly. Multi-omics analyses allow us to untangle these interactions and relationships, and in doing so provides us with a wider overview of what is going on at the biological level.
As well as being biologically interesting, insights from multi-omics data can support the development of more effective and personalized treatments. For instance, multi-omics analysis can help uncover novel drug targets and biomarkers as outlined in the case studies above, or improve patient stratification.
However, a word of caution: although multi-omics datasets can provide a great new depth of understanding, it’s not without a cost. Time, money, and skilled personnel are all required inputs to really get the most out of multi-omics datasets. That means that it’s important to ask yourself whether powerful multi-omics techniques are really required to answer your research question. Sometimes a simpler approach is actually sufficient.
If you decide that multi-omics analyses are necessary to answer your research question, it’s important to have a well-defined plan of action to make sure that you can truly get the most out of your multi-omics data. That’s why it’s generally recommended to get input from experts with a solid understanding of biostatistical methods to optimize its efficiency and prevent errors.
And that’s where BioLizard comes in! With our combined knowledge of biology and extensive hands-on experience in bioinformatics and analytics, including a proven track record in multi-omics analysis, we are ready to help you with all your multi-omics needs. Knowing that every multi-omics study is different, we tune our data analytical approaches to match your unique scientific goals. This is underlined by our fully customizable data visualization and exploration application, Bio|Mx, which allows you to effortlessly navigate across your multi-omics datasets to uncover new and actionable biological insights without needing any data science background.
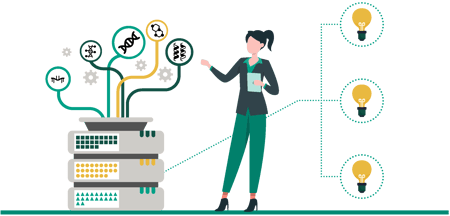
Are you hungry for more multi-omics information?
Stay tuned for the next blog in this series, in which we’ll outline our best practices for multi-omics analysis.
If you are ready to get started now on making your multi-omics analysis dreams a reality, reach out to us today!