Transforming your biomedical data into actionable insights
We are your agile data science partner with expertise in biomedical data analysis, data mining, data integration, pipeline development, machine learning and artificial intelligence, software engineering and more.
Who we work with
We support biotech, pharmaceutical and diagnostic companies, and research institutions of all sizes to enable truly data-driven discovery, preclinical, translational and clinical R&D processes. We also have robust experience working in the animal health field as well as in supporting food and agriculture R&D.
What we do
Leveraging our expertise in advanced bioinformatics, predictive analytics & AI, tailored software development, and data governance & architecture to support you in data-driven research and development and (pre)clinical studies.
BioLizard provided a highly professional and skilled deliverable to our request. The outcome and analysis of the hypothesis will help us in the decision-making process to identify an efficient strategy for further development of the drug product.
Tailored solutions
We help you make better, more data-driven decisions faster. Our goal? Supporting you in getting the most out of your biomedical data.
Bio|Verse® platform
We strongly believe that creating data-driven biological insights requires a combination of computational power, human input and collaboration.
How we do it
BioLizard brings together a diverse yet complementary range of expertises to implement the most up-to-date technological tools to meet your unique data needs, now and in the future. We believe that an inclusive and open communication strategy with our clients is essential for the successful completion of each project. We ensure to align regularly with you throughout the process, and adjust pipelines to best fit your unique data case and maximize your output.
When you partner with BioLizard, you get state-of-the-art data analysis pipelines and tools to apply to your current and future research projects - and moreover, you receive end-to-end support that will make it faster and easier for you to turn your data into actionable insights. We also always ensure to fully explain our data science approach to solving your biological challenges - there’s no black box when you work with BioLizard.
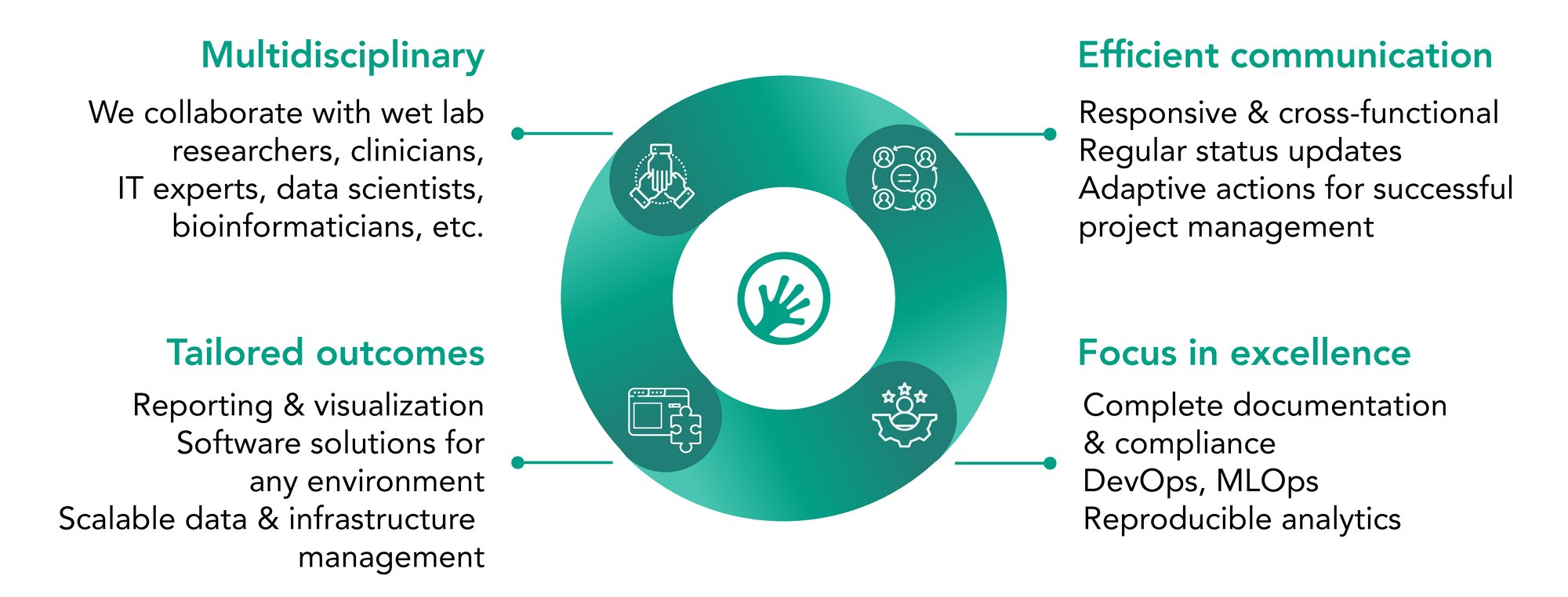
A personalized, agile service model for truly impactful results
No data? No worries!
The increasing availability of atlases and public datasets now gives scientists access to a wide variety of data derived from a range of different tissues and conditions - if you know where to find it and how to analyze it.
We can help you mine public data to find the answers you need - saving you time and money on data collection, and allowing you to directly form testable hypotheses based on data collected by others.
News & events
BioLizard Launches Bio|Verse® Navigator for Easy Exploration of Complex Biomedical Data
Ghent, (Belgium), December 5, 2024 - BioLizard, the bioinformatics, data engineering and solutions company for life sciences, today announced the launch of Bio|Verse Navigator, an innovative dashboard application developed to empower scientists in biotech, pharma, and healthcare to efficiently transform complex biomedical data into actionable insights. The application saves research and development time, supports seamless collaboration, and drives scientific discovery across human and animal health fields.
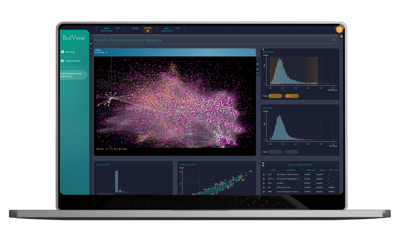
Watch our free webinar
Watch our in-depth webinar delving into the cutting-edge realm of spatial omics technologies.
In this webinar, experts from BioLizard and Pixelgen Technologies guide you through how spatial omics can deepen your biological understanding by preserving spatial context within molecular analyses.
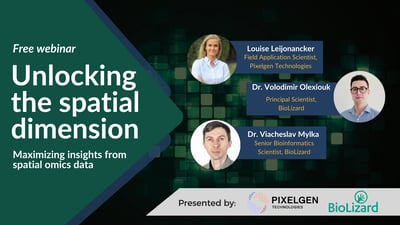
BioLizard featured on Hub & Spoken podcast
Volodimir Olexiouk, our Director of Scientific Engagement and Data Science Team Lead, was featured in episode 205 of Cynozure's Hub & Spoken podcast.
In this episode, host and CEO Jason Foster speaks with Volodimir about the revolutionary intersection of artificial intelligence and pharmaceuticals, exploring how AI is transforming the landscape of drug discovery.
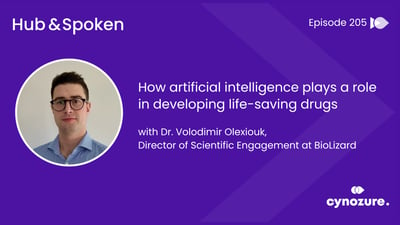
BioLizard announces Swiss subsidiary
We are proud to announce that we have made an exciting new step in our continued international expansion, while deepening our commitment to our current and future Swiss partners and clients.
With the opening of our newest office at Biopôle Lausanne, we have officially entered the Swiss market. Biopôle, which houses 120 companies, provides the perfect conditions for us to engage with the Swiss biotech industry and offer our services exactly where our clients need them.
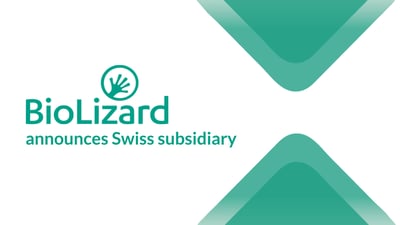
BioLizard and AbnomX announce partnership
We are happy to announce our strategic partnership with AbnomX.
BioLizard’s custom advanced analytics services complement AbnomX’s innovative, user-friendly platform for deciphering immune repertoire data.
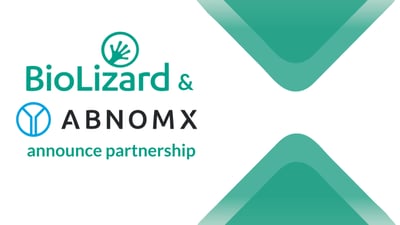
BioLizard featured on Data Science Leaders podcast
Volodimir Olexiouk, our Director of Scientific Engagement and Data Science Team Lead, was featured in episode 70 of Domino Data Lab's Data Science Leaders podcast.
In this episode, host Kjell Carlsson speaks with Volodimir about best practices for overcoming the data challenges for AI-driven drug discovery and combining scientific expertise with data science for augmented intelligence in the life sciences.
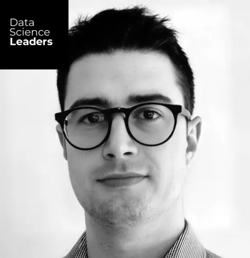
BioLizard Highlighted at Bio-EUROPE
"It's not trivial to make sure that you have your data (management) right, to then be able to apply advanced analytics."
MC Services interviewed our BD Director Amparo Cuéllar at Bio-EUROPE. Check out the 2-minute video wherein she discusses:
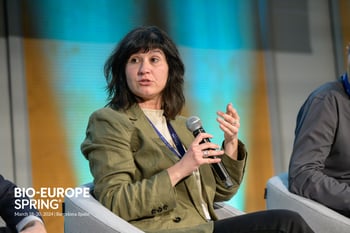