5 tips for getting the most out of your multi-omics data
In order to study a biological system as a whole, it’s beneficial to merge the input of different biological features. That means taking into account not only the proteome, transcriptome, and genome, but also the abundance of post-translational modifications and microbiome factors that can influence health and disease and impact ecosystems ranging from your gut to agricultural soil.
Alone, each of these features can provide an informative snapshot of how a biological system works. However, integrating all of these features provides a new depth of information, and a more unified picture of biological processes that occur within a cell, a tissue, or an ecosystem.
At BioLizard, we’ve invested a lot of time and effort into becoming true experts in multi-omics analysis, and have accumulated a track record of successful projects along the way. Based on this experience, we’ve come up with 5 top tips to consider when undertaking a multi-omics project - which we’ll share with you today!
But before we get to the best practices, let’s get familiar with one of the main challenges in multi-omics data analysis: data integration.
Data integration challenging but critical step for multi-omics analysis
Multi-omics data spans many dimensions - across both samples (hopefully - ideally we want a high n number) and across features of interest, such as genes, proteins, CpG sites, and more. This high dimensionality of data, or in other words the large amount of measured features per sample, is already a challenge in single omics studies, in which you might have a dataset encompassing a few dozen or even hundred samples, but thousands of genes per cell. However, this challenge looms even larger in multi-omics studies, in which a dataset encompassing hundreds of samples might not include only thousands of genes per sample, but also a great number of epigenomic modification sites and differentially expressed transcripts associated with each gene.
This challenge, known as the curse of dimensionality, can make it difficult to ensure that statistical analysis of your data is robust. As a result, it is important to devise a careful plan of action for which statistical methods you’ll employ to obtain reliable results, and to have a solid understanding of biology to make sure that the statistical output makes sense and is biologically relevant.
In fact, one important method of assessing whether data integration is successful is to check for a boost in the predictive capacity or clustering of integrated data versus that of single omics data. But in order to see if, for example, RNAseq data alongside CpG site methylation data provides a better predictive capacity for disease progression versus the RNAseq data alone, it helps to understand the biological system of interest. This is why it’s generally recommended to get input from experts with a solid understanding of biostatistical methods - ideally from the very start of your multi-omics study - to optimize efficiency and prevent errors.
So, what are our in-house multi-omics experts’ best practices for tackling the challenges that come with multi-omics data integration? Let’s dive in.
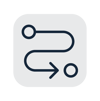
Start at the end: What do you want to get out of your multi-omics data analysis?
When you’re starting out, it’s important to think about your end-game.
Multi-omics studies can provide incredible insight into biological processes, but to uncover those insights, the input needs to be of a high quality. To achieve this, careful planning and execution are essential in the sample preparation stage.
Before you begin in the wet lab, you should precisely frame the exact research question that you want to answer, define clear hypotheses, and then design your study accordingly. When designing your study, it’s important to consider questions like,
- “Will my samples be measured all at once, or over different time points?”
- “Will my samples be measured individually, or pooled?”
- “What is the control for my intervention of interest?”
- “What type of sample(s) am I using?”
The answers to these questions can impact the robustness, power, and necessary statistical tests to answer your overarching research question. When devising your plan of action accordingly, it can help to have an experienced bioinformatician involved to make sure you’re setting yourself up for success - like the team at BioLizard!
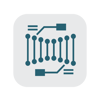
Choose the right sequencing platforms
When you’re starting out, it’s also important to select the right sequencing platforms. These days, there is an abundance of different omics technologies targeting DNA, total RNA, mRNA, miRNA, DNA methylation, proteins, protein modifications, histone post-translational modifications, metagenomics, metaproteomics, and more. The challenge isn’t to find technologies that can deal with your biological levels of interest - it’s finding the right ones to match your biological questions.
For instance, take genomics. DNA sequencing technologies tend to balance error rates and read lengths, and you’ll need to make a choice about what to prioritize depending on the research question you want to answer.
For example, while Illumina short read sequencing technologies have a maximum read length of around 600 bases, they typically have very low error rates of only about 0.25% per base. The downside? These technologies, which include HiSeq and MiniSeq, are sensitive to low diversity libraries. On the other hand, long-read technologies like PacBio and Oxford Nanopore instruments have higher error rates - up to 15 or even 20% per base respectively - but can sequence a whopping 30 kilobases in a single read. Nanopore sequencing has even been reported to reach a record read length of 2.3 megabases.
It’s also important to understand the biological background of your samples when using proteomics technologies. For instance, if you’re interested in a low-abundance protein type, you might have to take an extra step of removing high-abundance proteins from your sample. For more in-depth information, we recommend that you listen to our recent podcast on proteomics.
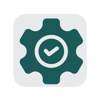
Quality control is essential in multi-omics analysis
Quality control is a fundamental step in multi-omics data analysis - especially when leveraging public data. It’s an unfortunate reality that public data can sometimes be of poor quality. So, to make sure that you’re only integrating high-quality data to ultimately achieve clinically relevant and biologically accurate insights, it’s important to select only good quality (meta)data for inclusion in your study.
Alongside the importance of quality control while using public datasets, it’s also important to consider the features of different omics platforms used when integrating data - whether it’s public or in-house. Different omics platforms have different signal-to-noise ratios and confer differing statistical powers, and there’s always a possibility of confounding and technical artifacts leaking into your data. That’s why careful attention always needs to be paid to data interpretation, even if you’ve been careful to select the best sequencing platforms for your research question.
In a perfect world, careful selection of different individual omics platforms would be done at the experimental design stage, but that’s not always possible - and you don’t need to let it stop you from integrating historical datasets that have been sitting in your metaphorical filing cabinets. You can definitely use historical data to uncover new insights - but even if you’ve already collected all the data you want to use, it can still be helpful to involve an experienced bioinformatician to make sure that data normalisation and filtering are performed properly.
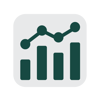
Choose the right methods for multi-omics integration and analysis
OK, so you’ve performed quality control, and you’re ready to start integrating your omics datasets! But wait… which integration method should you use?
At this stage, you have a few options, and there are pros and cons to all of the different integration methods. Your choice will depend on the biological question that needs to be answered, alongside practical details regarding sample preparation, sample quality, and sequencing platform used.
For example, you will need to choose whether you want to apply multi-staged or meta-dimensional integration of your data. Multi-staged integration models use multiple steps to combine two feature types of your data at a time, for instance gene expression counts from an RNASeq experiment together with protein intensity data originating from a mass spectrometry experiment, and then subsequently unravel associations between those different data types and your phenotype of interest. On the other hand, meta-dimensional approaches attempt to incorporate all data types of interest simultaneously. Both types of integration work well - the question is rather what will work best for your particular datasets and biological questions.
After integrating your data, it’s also important to check if the integration was successful. As mentioned before, a common way of doing this is to check for a boost in predictive capacity of the integrated datasets, versus that of the individual single omics datasets. In addition, if you’re using any new methodologies, it’s important to benchmark them against known and published methodologies, using trusted datasets. This is a key task for ensuring that fundamental pillar of science: repeatability!
Data integration and analysis often presents a challenge to R&D specialists because most data integration and analysis tools still require a bioinformatics background. On top of that, there are a lot of different available packages for crunching multi-omics data to choose from, and all of them have slightly different capabilities. To get the most out of your data, it’s important to choose the right one. It’s also often key to tailor statistical analysis to your specific research project and question, with a mind for the underlying statistical assumptions and any unique limitations in your datasets.
And this is where BioLizard can come in! We strive to achieve perfect repeatability within our analyses and processes to ensure that results are accurate, and we have a track-record of developing tailored, best-in-class methodology to deal with a wide range of unique multi-omics projects. And on top of that, we have built a uniquely user-friendly and modular data analysis and exploration application for turning multi-omics data into actionable insights…
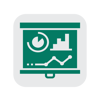
Use powerful visualization tools to extract information from multi-omics data
Which brings us to our last tip: to use a data visualization and exploration tool that allows you to interact intimately with your multi-omics data, and harvest knowledge directly from your results.
At BioLizard, we have built Bio|Mx: a fully customizable data visualization and exploration application that allows you to effortlessly navigate across your multi-omics datasets - which stay safe and secure in a closed cloud environment.
Bio|Mx is specifically designed to accomplish integration and analysis of complex multi-omics data from both public and your own proprietary omics datasets, by leveraging sophisticated deep learning algorithms to help you reach comprehensive overviews of your biological systems of interest. Our goal is to help you explore interactions that emerge from multi-omics analyses through a user-friendly interface that doesn’t require any coding knowledge. This, combined with simple export of results and reports, empowers you to explore your own data interactively, identify trends, and formulate testable, data-driven hypotheses. If you’re interested in learning more, find more here or reach out to us today.
Making the most of your multi-omics data analysis
Multi-omics is a powerful tool to answer biological questions and uncover clinically relevant insights - but it’s important to ask yourself the right questions along the way to get the most out of a multi-omics project. As one publication put it, expert knowledge can be valuable at any stage of a multi-omics project to optimize the study and prevent errors and resource waste.
So, are you ready to get started?
Reach out to BioLizard today!